top of page
3D Object Detection

Image-to-Lidar Relational Distillation for Autonomous Driving Data
Anas Mahmoud, Ali Harakeh, Steven L Waslander
European Conference on Computer Vision (ECCV), 2024.
We estimate the similarity between negative samples in the representation space of self-supervised vision models to improve contrastive learning.

Self-Supervised Image-to-Point Distillation via Semantically Tolerant Contrastive Loss
Anas Mahmoud, Jordan SK Hu, Tianshu Kuai, Ali Harakeh, Liam Paull, Steven L Waslander
Proceedings of the IEEE conference on computer vision and pattern recognition (CVPR), 2023
We identify self-similarity and severe class imbalance as two major challenges in self-supervised image-to-point representation learning for autonomous driving datasets.
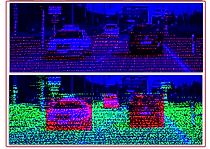
DVF: Dense Voxel Fusion for 3D Object Detection
Anas Mahmoud, and Steven L. Waslander
Proceedings of the IEEE/CVF Winter Conference on Applications of Computer Vision (WACV), 2023
we propose Dense Voxel Fusion (DVF), a sequential fusion method, that first assigns voxel centers to the 3D location of the occupied LiDAR voxel features.

PDV: Point Density-Aware Voxels for LiDAR 3D Object Detection
Jordan S.K. Hu, Tianshu Kuai and Steven L. Waslander
Proceedings of the IEEE conference on computer vision and pattern recognition (CVPR), 2022
We propose a Point Density-Aware Voxel network (PDV) to resolve LiDAR’s diverging point pattern issues by leveraging voxel point centroid localization and feature encodings. ​

Bayesian Embeddings for Few-Shot Open World Recognition
John Willes, James Harrison, Ali Harakeh, Chelsea Finn, Marco Pavone, and Steven Waslander
IEEE Transactions on Pattern Analysis and Machine Intelligence (T-PAMI), 2022 ​
We combine Bayesian non-parametric class priors with an embedding-based scheme to yield a highly flexible framework which we refer to as few-shot learning for open world recognition (FLOWR).. ​
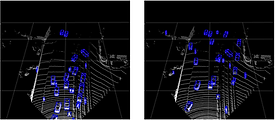
Pattern-Aware Data Augmentation for LiDAR 3D Object Detection
Jordan S.K. Hu and Steven L. Waslander
IEEE International Intelligent Transportation Systems Conference (ITSC), 2021 ​
Data augmentation technique that uses the diverging LiDAR point pattern to simulate objects at farther distances. ​
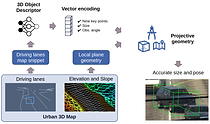
UrbanNet: Leveraging Urban Maps for Long Range 3D Object Detection
Juan Carrillo, and Steven L. Waslander
IEEE International Intelligent Transportation Systems Conference (ITSC), 2021 ​
We present UrbanNet, a modular architecture for long range monocular 3D object detection with static cameras. ​
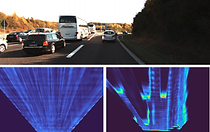
Categorical Depth Distribution Network for Monocular 3D Object Detection
Cody Reading, Ali Harakeh, Julia Chae, Steven L. Waslander
IEEE/CVF Conference on Computer Vision and Pattern Recognition (CVPR, Oral), 2021
​
Monocular 3D object detection pipeline that estimates pixel-wise categorical depth distributions to accurately locate image information in 3D space.

CG Stereo: Confidence Guided Stereo 3D Object Detection with Split Depth Estimation
Chengyao Li, Jason Ku, and Steven L. Waslander
IEEE/RSJ International Conference on Intelligent Robots and Systems (IROS), 2020
Confidence-guided stereo 3D object detection pipeline that uses separate decoders for foreground and background pixels during depth estimation
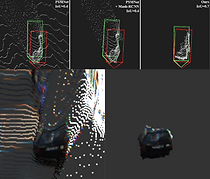
OC Stereo: Object-Centric Stereo Matching for 3D Object Detection
​
Alex D. Pon, Jason Ku, Chengyao Li, and Steven L. Waslander
International Conference on Robotics and Automation (ICRA), 2020
Object-centric stereo matching module that focuses on predicting the disparities of objects of interest to remove streaking artifacts.
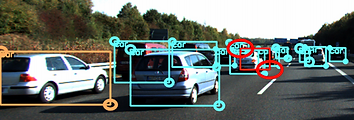
BayesOD: A Bayesian Approach for Uncertainty Estimation in Deep Object Detectors
Ali Harakeh, Michael Smart, Steven L. Waslander
International Conference on Robotics and Automation (ICRA), 2020
​
An uncertainty estimation approach that reformulates the standard object detector inference and Non-Maximum suppression components from a Bayesian perspective.
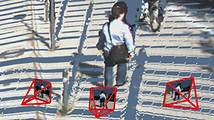
VMVS: Improving 3D Object Detection for Pedestrians with Virtual Multi-View Synthesis
​
Jason Ku, Alex D. Pon, Sean Walsh, and Steven L. Waslander
IEEE/RSJ International Conference on Intelligent Robots and Systems (IROS), 2019
​
In this work, we presnet a virtual multi-view synthesis module for improving orientation estimation of pedestrians.

MonoPSR: Monocular 3D Object Detection Leveraging Accurate Proposals and Shape Reconstruction
Jason Ku*, Alex D. Pon*, and Steven L. Waslander
IEEE/CVF Conference on Computer Vision and Pattern Recognition (CVPR), 2019
Monocular 3D object detection method that uses proposals and leverages shape reconstruction. Can be found on the KITTI benchmark under MonoPSR.
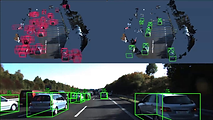
AVOD:Joint 3D Proposal Generation and Object Detection from View Aggregation
Jason Ku, Melissa Mozifian, Jungwook Lee, Ali Harakeh, and Steven L. Waslander
IEEE/RSJ International Conference on Intelligent Robots and Systems (IROS), 2018
​
we propose a novel feature extractor that produces high resolution feature maps from LIDAR point clouds and RGB images, allowing for the localization of small classes in the scene.
bottom of page